Server Specifications
Server Configuration:
- Price: $409.00/month
- CPU: Dual 18-Core E5-2697v4 (36 cores, 72 threads)
- RAM: 256GB
- Storage: 240GB SSD + 2TB NVMe + 8TB SATA
- Network: 100Mbps-1Gbps connection
- OS: Windows 11 Pro
- Software: Ollama versions 0.5.4
GPU Details:
- GPU: Nvidia GeForce RTX 4090
- Compute Capability: 8.9
- Microarchitecture: Ada Lovelace
- CUDA Cores: 16,384
- Tensor Cores: 512
- GPU Memory: 24GB GDDR6X
- FP32 Performance: 82.6 TFLOPS
With 82.6 TFLOPS FP32 performance, 16,384 CUDA cores, and 512 Tensor cores, the NVIDIA RTX 4090 outshines most consumer-grade GPUs in both compute capability and cost-efficiency.
LLMs Reasoning Tested on Ollama with RTX 4090
- LLaMA Series: LLaMA 2 (13B), LLaMA 3.1 (8B)
- Qwen Series: Qwen (14B, 32B)
- Phi Series: Phi4 (14B)
- Mistral Models: Mistral-small (22B)
- Falcon Series: Falcon (40B)
- Gemma and LLaVA: Gemma2 (27B), LLaVA (34B)
Benchmark Results: Ollama GPU RTX 4090 Performance Metrics
Models | deepseek-r1 | deepseek-r1 | llama2 | llama3.1 | qwen2.5 | qwen2.5 | gemma2 | phi4 | qwq | llava |
---|---|---|---|---|---|---|---|---|---|---|
Parameters | 14b | 32b | 13b | 8b | 14b | 32b | 27b | 14b | 32b | 34b |
Size | 9 | 20 | 7.4 | 4.9 | 9 | 20 | 16 | 9.1 | 20 | 19 |
Quantization | 4 | 4 | 4 | 4 | 4 | 4 | 4 | 4 | 4 | 4 |
Running on | Ollama0.5.7 | Ollama0.5.7 | Ollama0.5.4 | Ollama0.5.4 | Ollama0.5.4 | Ollama0.5.4 | Ollama0.5.4 | Ollama0.5.4 | Ollama0.5.4 | Ollama0.5.4 |
Downloading Speed(mb/s) | 12 | 12 | 12 | 12 | 12 | 12 | 12 | 12 | 12 | 12 |
CPU Rate | 2% | 3% | 1% | 2% | 3% | 3% | 2% | 3% | 2% | 2% |
RAM Rate | 3% | 3% | 3% | 3% | 3% | 3% | 3% | 3% | 3% | 3% |
GPU vRAM | 45% | 90% | 41% | 65% | 45% | 90% | 78% | 47% | 90% | 92% |
GPU UTL | 95% | 98% | 92% | 94% | 96% | 97% | 96% | 97% | 99% | 97% |
Eval Rate(tokens/s) | 58.62 | 34.22 | 70.90 | 95.51 | 63.92 | 34.39 | 37.97 | 68.62 | 31.80 | 36.67 |
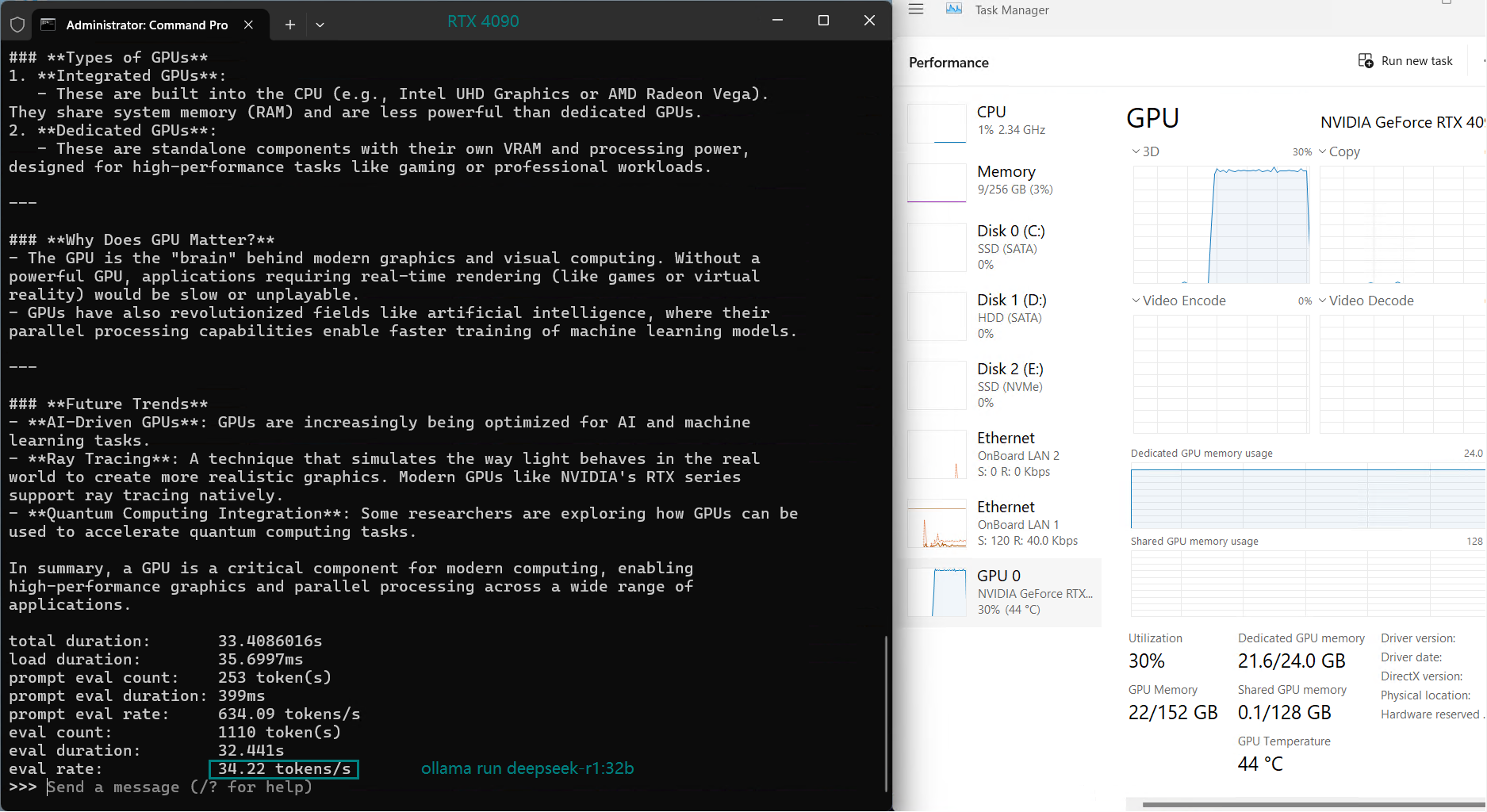
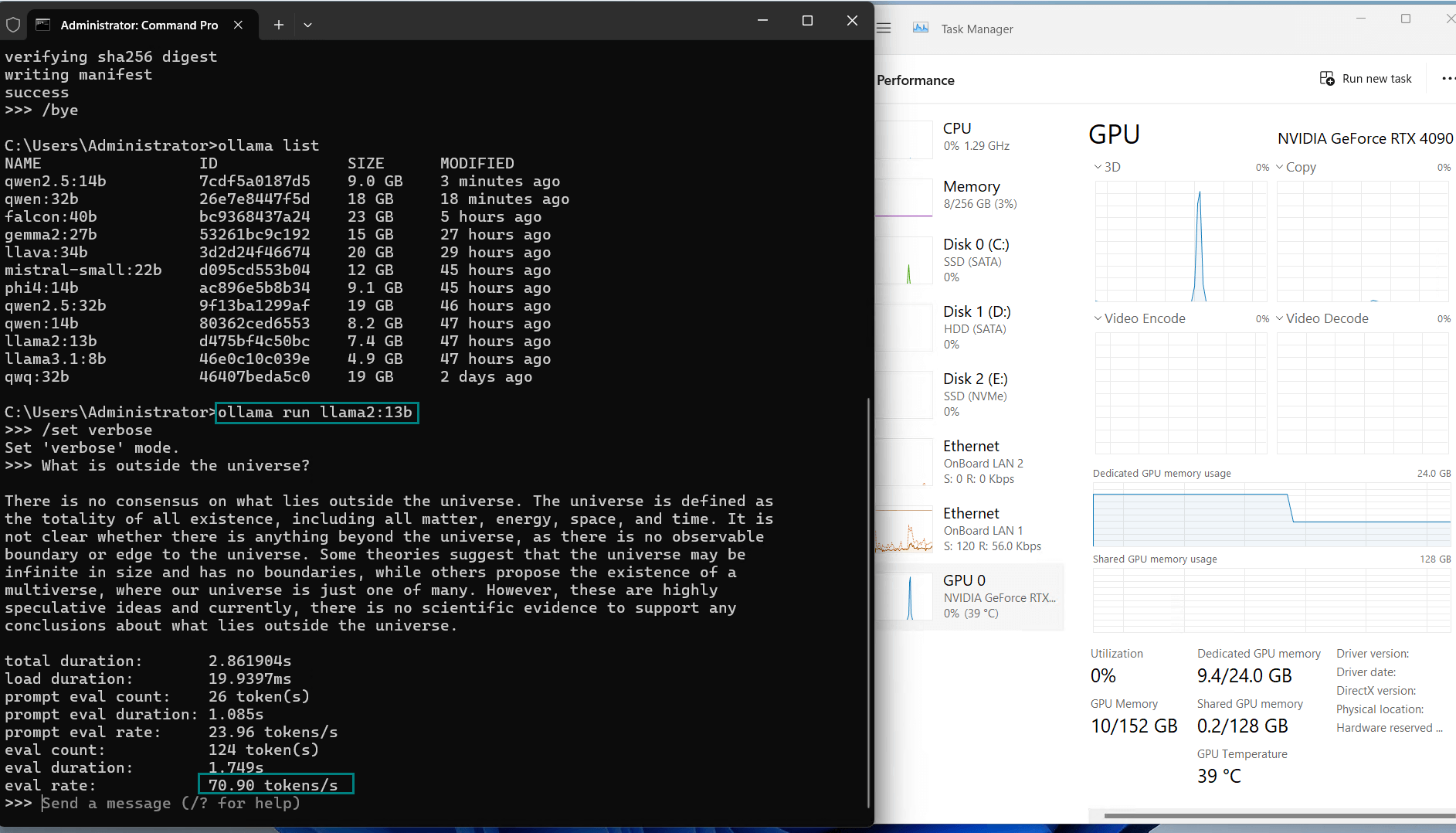
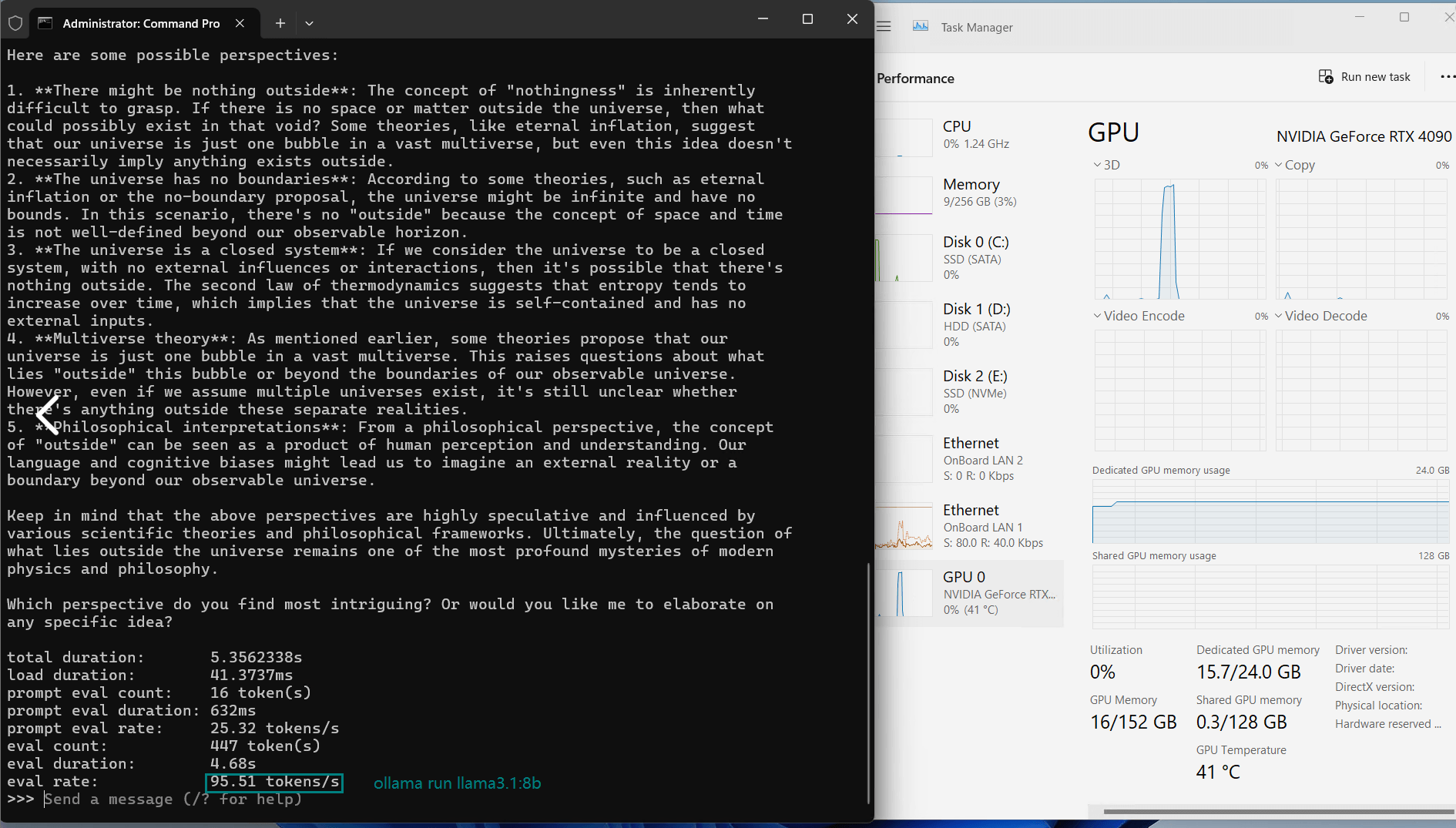
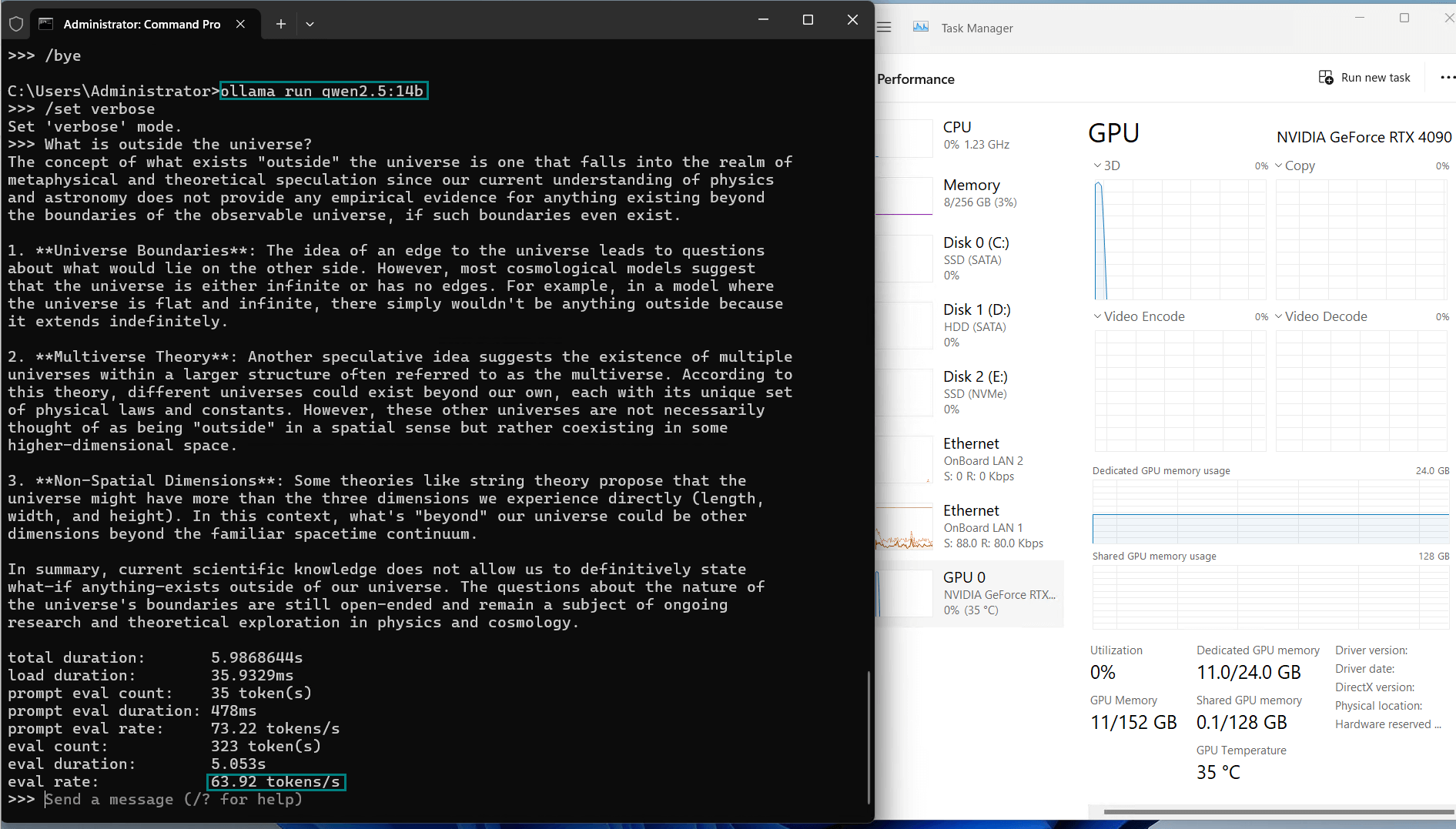
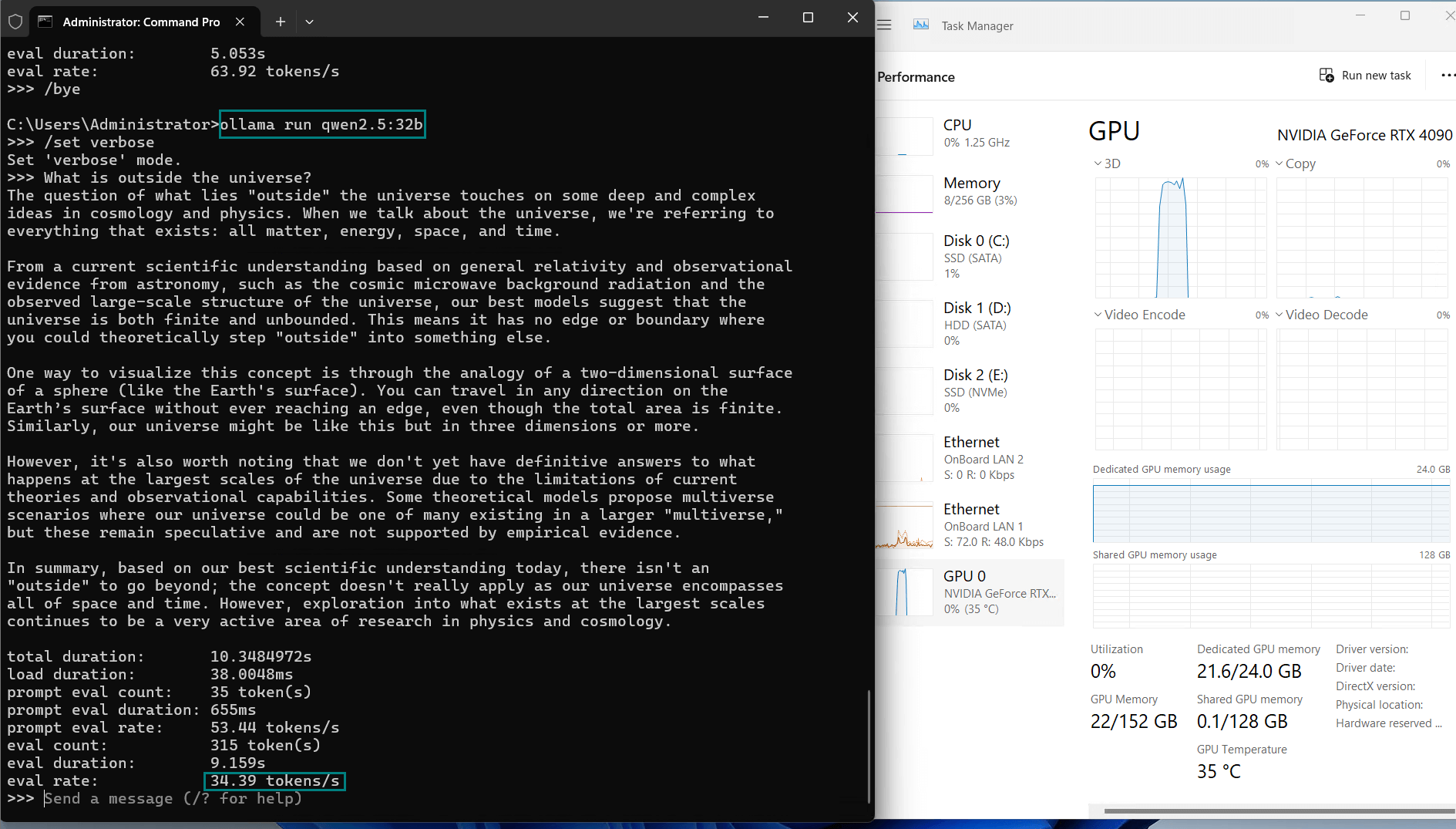
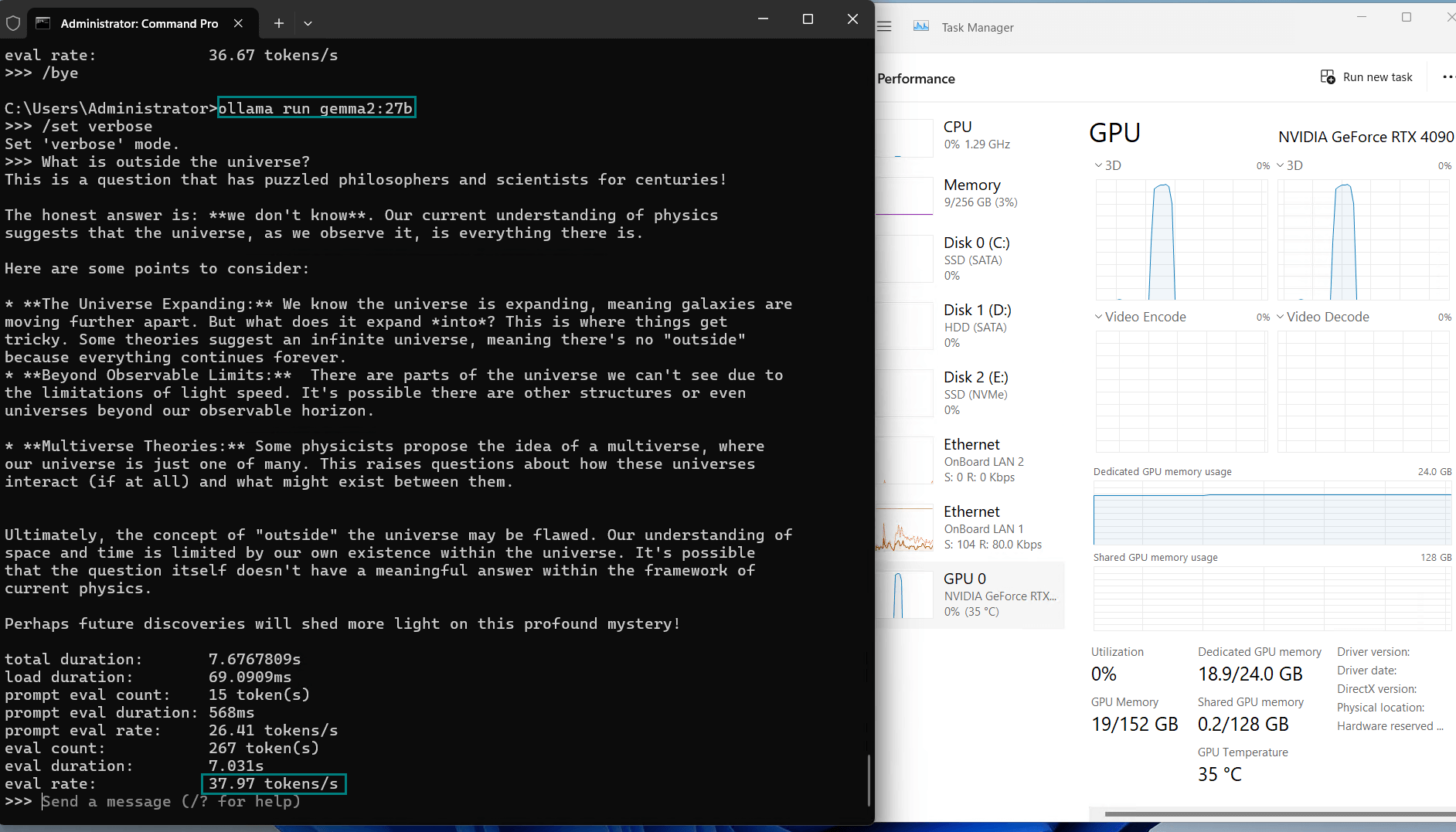
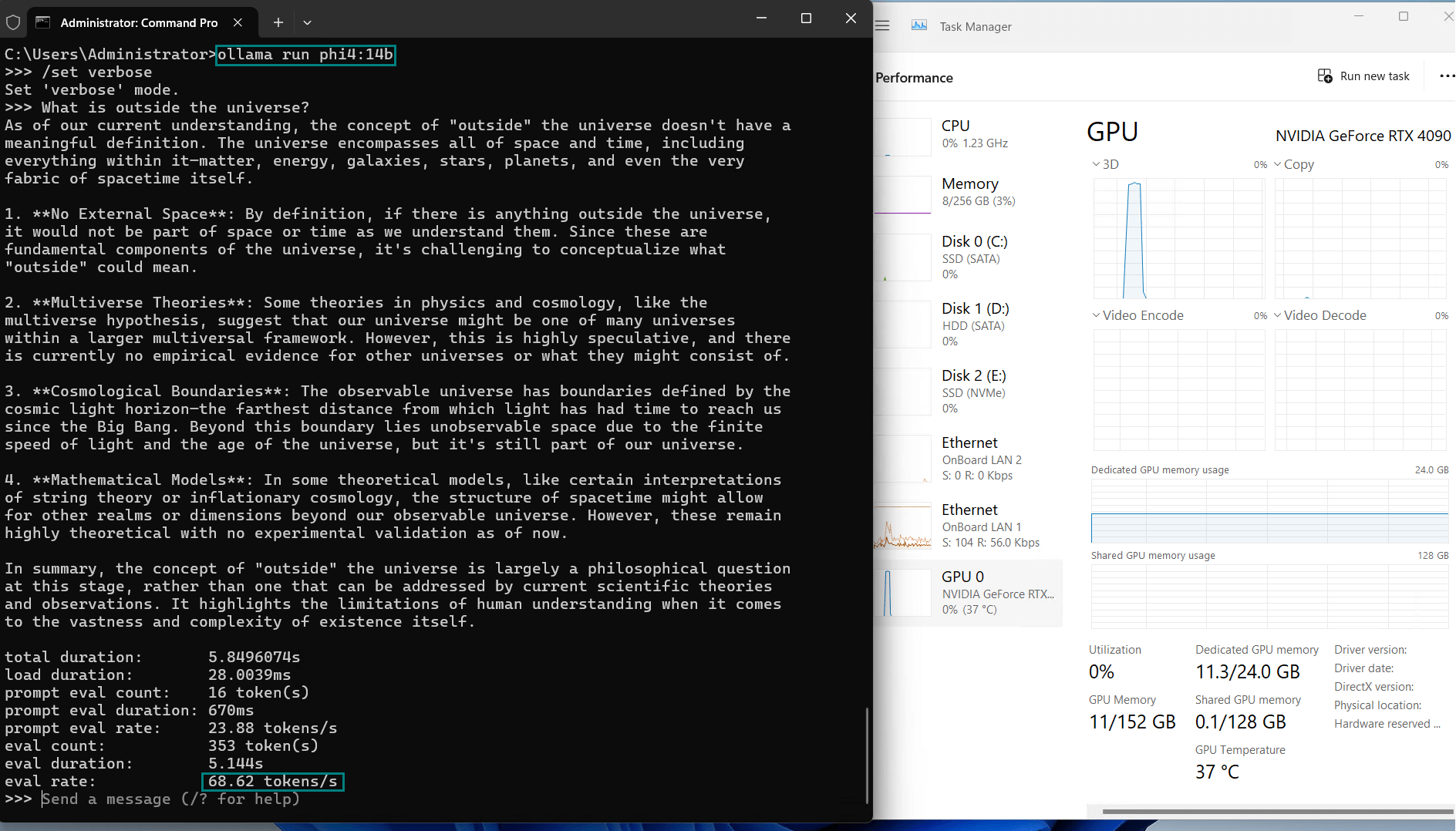
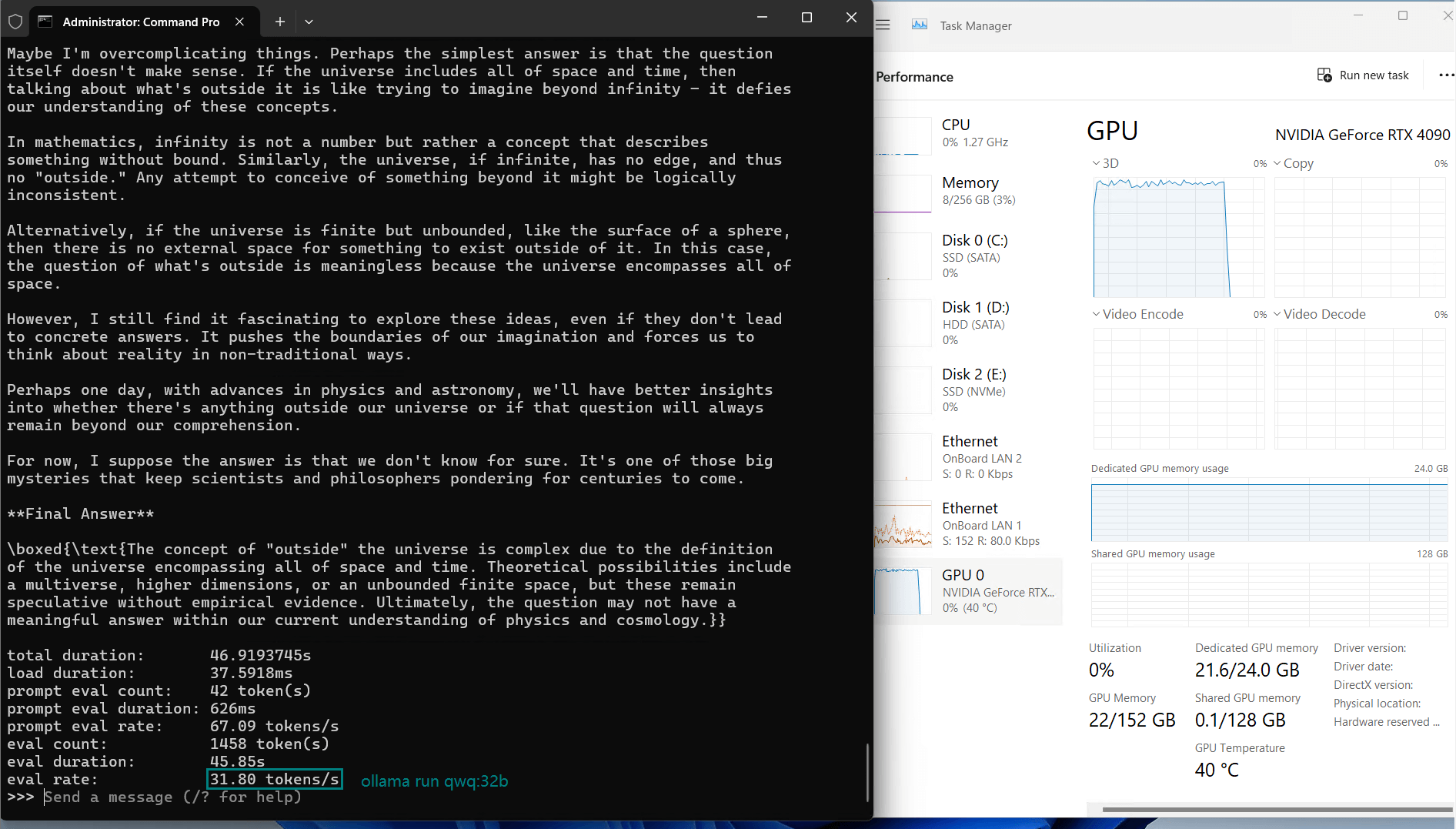
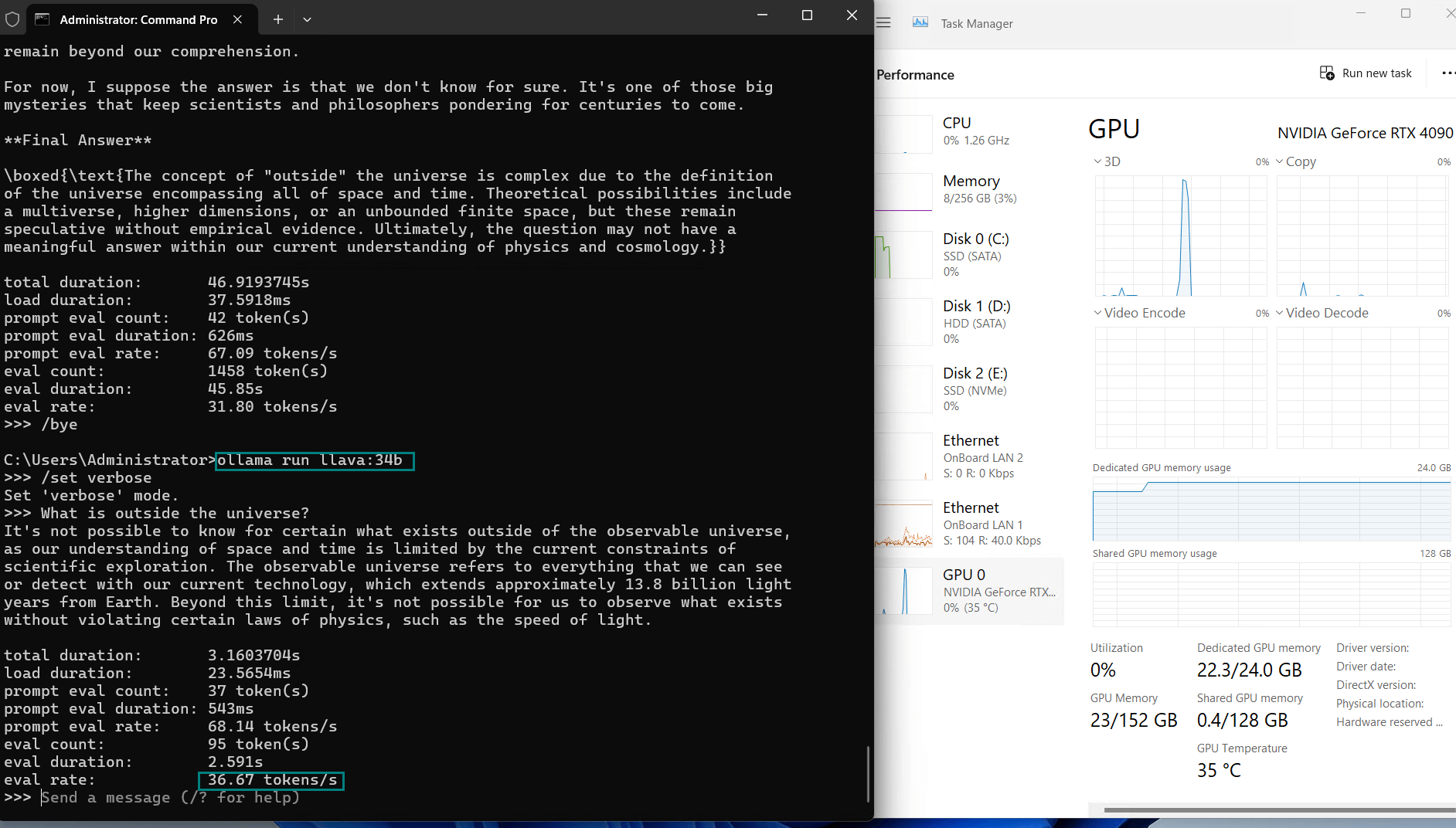
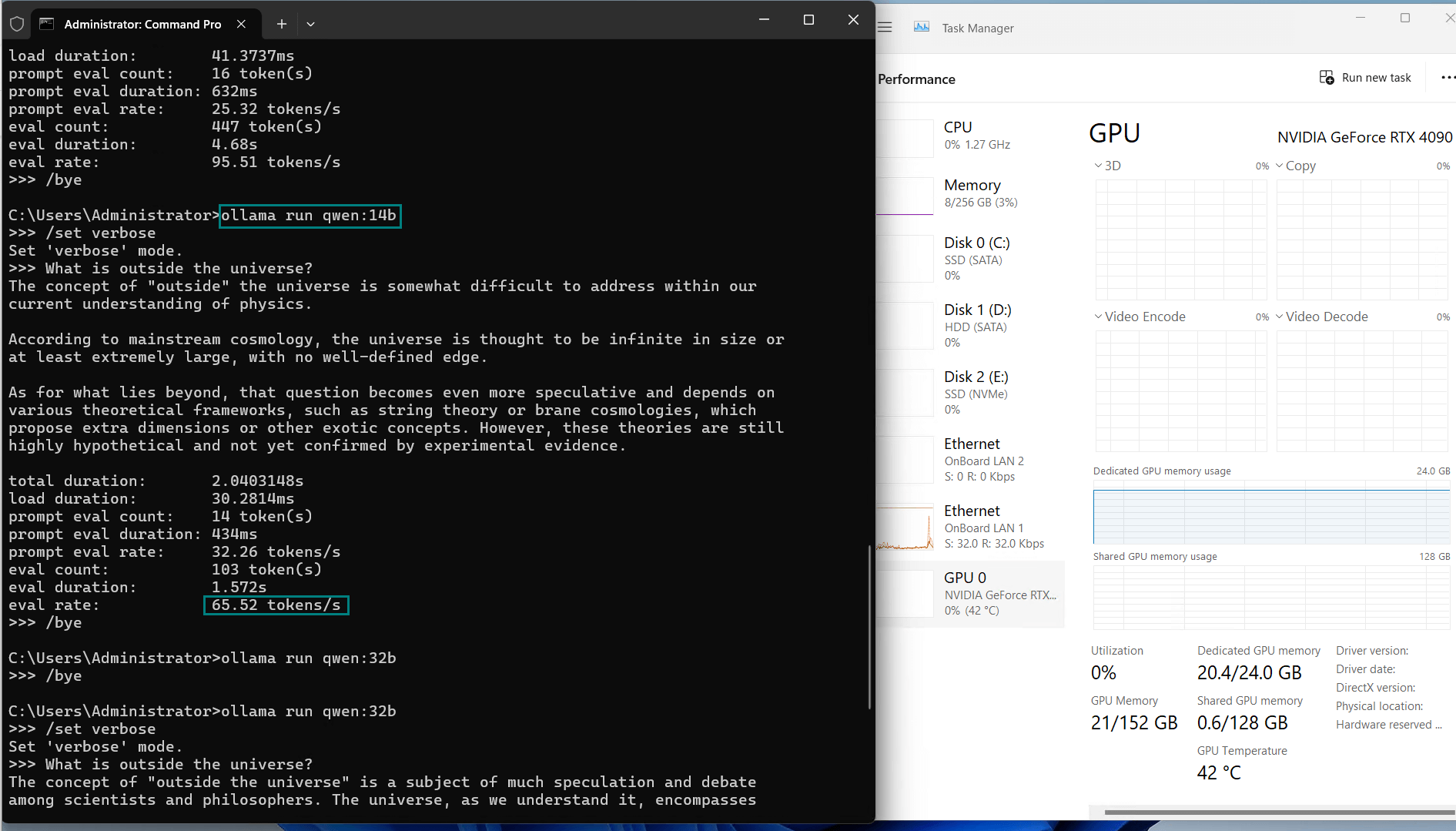
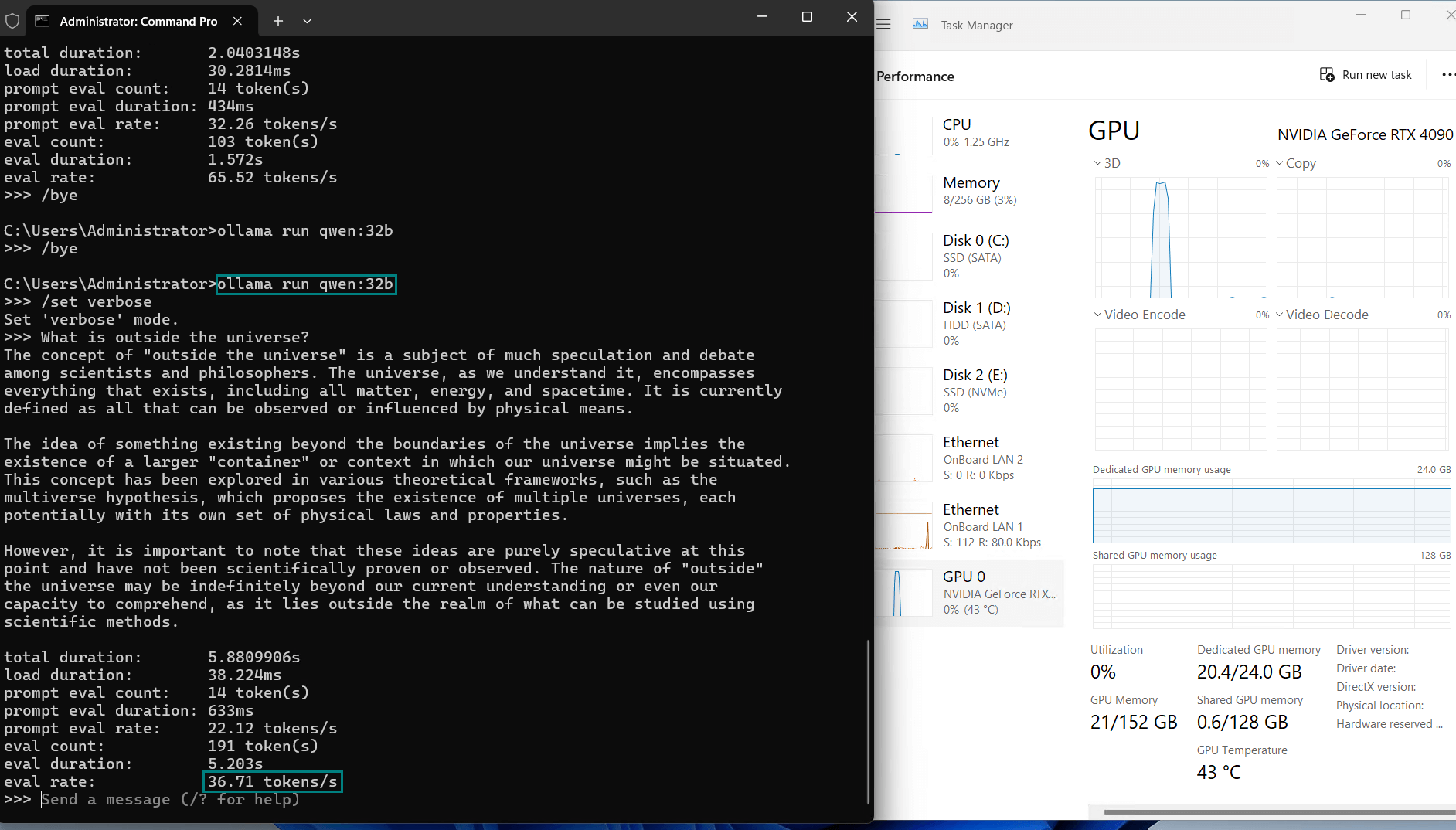
Key Insights
1. Small to Mid-Sized Models (8B-34B)
2. Not Enough for 40B Models
3. Cost-Effective
Metric | Value for Various Models |
---|---|
Downloading Speed | 12 MB/s for all models, 118 MB/s When a 1gbps bandwidth add-on ordered. |
CPU Utilization Rate | Maintain 1-3% |
RAM Utilization Rate | Maintain 2-4% |
GPU vRAM Utilization | 41-92%. The larger the model, the higher the utilization rate. |
GPU Utilization | 92%+. Maintain high utilization rate. |
Evaluation Speed | 30+ tokens/s. It is recommended to use models below 36b. |
Performance Comparison with Other Graphics Cards
Enterprise GPU Dedicated Server - RTX 4090
- 256GB RAM
- Dual 18-Core E5-2697v4
- 240GB SSD + 2TB NVMe + 8TB SATA
- 100Mbps-1Gbps
- OS: Windows / Linux
- GPU: GeForce RTX 4090
- Microarchitecture: Ada Lovelace
- CUDA Cores: 16,384
- Tensor Cores: 512
- GPU Memory: 24 GB GDDR6X
- FP32 Performance: 82.6 TFLOPS
- Perfect for 3D rendering/modeling , CAD/ professional design, video editing, gaming, HPC, AI/deep learning.
Enterprise GPU Dedicated Server - RTX A6000
- 256GB RAM
- Dual 18-Core E5-2697v4
- 240GB SSD + 2TB NVMe + 8TB SATA
- 100Mbps-1Gbps
- OS: Windows / Linux
- GPU: Nvidia Quadro RTX A6000
- Microarchitecture: Ampere
- CUDA Cores: 10,752
- Tensor Cores: 336
- GPU Memory: 48GB GDDR6
- FP32 Performance: 38.71 TFLOPS
- Optimally running AI, deep learning, data visualization, HPC, etc.
Enterprise GPU Dedicated Server - A100
- 256GB RAM
- Dual 18-Core E5-2697v4
- 240GB SSD + 2TB NVMe + 8TB SATA
- 100Mbps-1Gbps
- OS: Windows / Linux
- GPU: Nvidia A100
- Microarchitecture: Ampere
- CUDA Cores: 6912
- Tensor Cores: 432
- GPU Memory: 40GB HBM2
- FP32 Performance: 19.5 TFLOPS
- Good alternativeto A800, H100, H800, L40. Support FP64 precision computation, large-scale inference/AI training/ML.etc
Multi-GPU Dedicated Server- 2xRTX 4090
- 256GB RAM
- Dual 18-Core E5-2697v4
- 240GB SSD + 2TB NVMe + 8TB SATA
- 1Gbps
- OS: Windows / Linux
- GPU: 2 x GeForce RTX 4090
- Microarchitecture: Ada Lovelace
- CUDA Cores: 16,384
- Tensor Cores: 512
- GPU Memory: 24 GB GDDR6X
- FP32 Performance: 82.6 TFLOPS
Summary and Recommendations
The NVIDIA RTX 4090 benchmark showcases its exceptional ability to handle LLM inference workloads, particularly for small-to-medium models. Paired with Ollama, this setup provides a robust, cost-effective solution for developers and enterprises seeking high performance without breaking the bank.
However, for larger models and extended scalability, GPUs like the NVIDIA A100 or multi-GPU configurations might be necessary. Nonetheless, for most practical use cases, the RTX 4090 hosting solution is a game-changer in the world of LLM benchmarks.
RTX 4090, Ollama, LLMs, AI benchmarks, GPU performance, NVIDIA GPU, AI hosting, 4-bit quantization, Falcon 40B, LLaMA 2, GPU server, machine learning, RTX 4090 benchmark, Ollama benchmark, NVIDIA GPU, LLM performance, GPU hosting, 4-bit quantization, Falcon 40B, LLaMA 2 benchmark, AI model hosting, GPU server performance